By incorporating AI agents into your workflow, you can greatly enhance productivity and efficiency. AI-driven strategies like task prioritization, dynamic resource allocation, and intelligent routing can reduce project delivery times by up to 25% and increase productivity by 40%. Real-time monitoring and feedback loops facilitate continuous improvement, while AI-powered automation and orchestration offload repetitive tasks. You can also utilize human-AI collaboration, AI-powered analytics, and adaptive task scheduling to refine your workflow. As you investigate these strategies, you'll uncover more opportunities to optimize your task workflow and access even greater efficiencies.
Need-to-Knows
- AI agents prioritize tasks based on urgency, importance, and required skills, reducing project delivery times by up to 25%.
- Real-time data analysis optimizes task assignments, predicting demand fluctuations and enabling adaptive resource distribution.
- Intelligent agents automate repetitive tasks, enhancing workflow efficiency and reducing human error in data-intensive tasks.
- Continuous learning cycles refine AI decision-making through real-time feedback, anticipating potential bottlenecks and maintaining operational efficiency.
- Machine learning models analyze task completion patterns and resource availability, reducing idle time and boosting productivity by 20-30%.
Task Prioritization With AI Agents
Most organizations face the challenging endeavor of managing multiple projects simultaneously, and ineffective task prioritization can lead to missed deadlines, wasted resources, and decreased productivity.
To overcome this, you can utilize AI agents to optimize task prioritization. These agents utilize algorithms to assess task urgency and importance, enabling them to prioritize tasks effectively based on predefined criteria such as deadlines, resource availability, and potential impact.
By analyzing historical data, AI agents can predict task completion times and prioritize accordingly, resulting in a 20-30% increase in overall workflow efficiency. Furthermore, they can dynamically adjust task priorities in real-time by accessing current data inputs, ensuring that the most critical tasks are addressed swiftly, even in changing environments.
Incorporating machine learning techniques allows AI agents to learn from past decision-making patterns, improving their prioritization strategies over time and leading to better outcomes in task management.
Dynamic Resource Allocation Strategies
As you endeavor to optimize your task workflow, dynamic resource allocation strategies emerge as a crucial component, allowing you to maximize productivity and efficiency.
By leveraging real-time data analysis, you can optimize task assignments based on agent availability, workload, and skill sets, leading to improved overall efficiency. Machine learning algorithms can predict demand fluctuations, permitting you to adjust resource distribution accordingly, which can result in a potential 20-30% increase in productivity.
Implementing AI agents for dynamic resource allocation allows 24/7 operational capability, ensuring tasks are managed without downtime and resources are utilized effectively across different time zones.
Furthermore, feedback loops can be implemented, allowing AI agents to learn from past performance, leading to continuous improvement in resource allocation strategies and markedly reducing response times by 15-25%.
In complex environments, multi-agent systems can collaborate to dynamically allocate resources, facilitating improved decision-making and faster task completion rates, which can improve customer satisfaction by up to 40%.
Intelligent Task Routing and Escalation
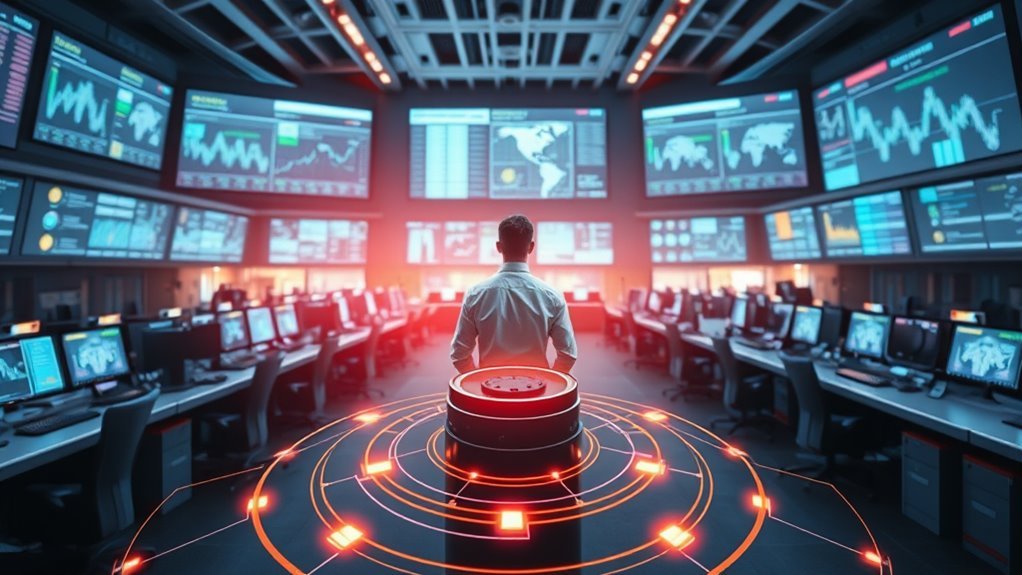
Your task workflow optimization journey now takes you to the domain of intelligent task routing and escalation, where AI-driven strategies revolutionize the way tasks are assigned and managed.
By leveraging intelligent agents, you can optimize task allocation, guaranteeing that each task is routed to the most suitable agent based on their skills and availability. This approach leads to a notable 30% increase in task resolution efficiency.
Escalation protocols are likewise integrated into the system, automatically elevating tasks that remain unresolved beyond specific time thresholds. This guarantees that critical issues receive timely attention, preventing potential customer dissatisfaction.
AI-driven systems continuously improve routing accuracy by incorporating historical data and machine learning, adapting to changing workloads and agent performance metrics over time.
By implementing intelligent task routing, you can reduce average handling time (AHT) by up to 20%, considerably improving overall productivity and service levels.
Effective escalation strategies improve customer satisfaction and optimize resource allocation within the workflow, allowing agents to focus on high-priority tasks.
Real-Time Task Monitoring and Feedback
With intelligent task routing and escalation in place, you're now poised to access the full potential of your workflow through utilizing the capabilities of real-time task monitoring and feedback.
By implementing real-time monitoring, you can continuously track task performance and make immediate adjustments based on current data. This is made possible through feedback loops that collect user input and performance metrics, improving the adaptability and learning capabilities of AI agents over time.
Here's what you can expect from real-time task monitoring and feedback:
- Identify bottlenecks and inefficiencies in your workflow using analytics tools, enabling proactive problem-solving and improved workflow efficiency.
- Automate the evaluation of completed tasks, providing instant feedback that enriches decision-making and supports continuous improvement.
- Guarantee AI agents remain aligned with business objectives, maximizing operational effectiveness and responsiveness.
- Utilize real-time monitoring to facilitate continuous improvement, driving adaptability and responsiveness in your workflow.
- Improve task performance through data-driven insights, leading to increased workflow efficiency and reduced bottlenecks.
AI-Driven Task Automation and Orchestration
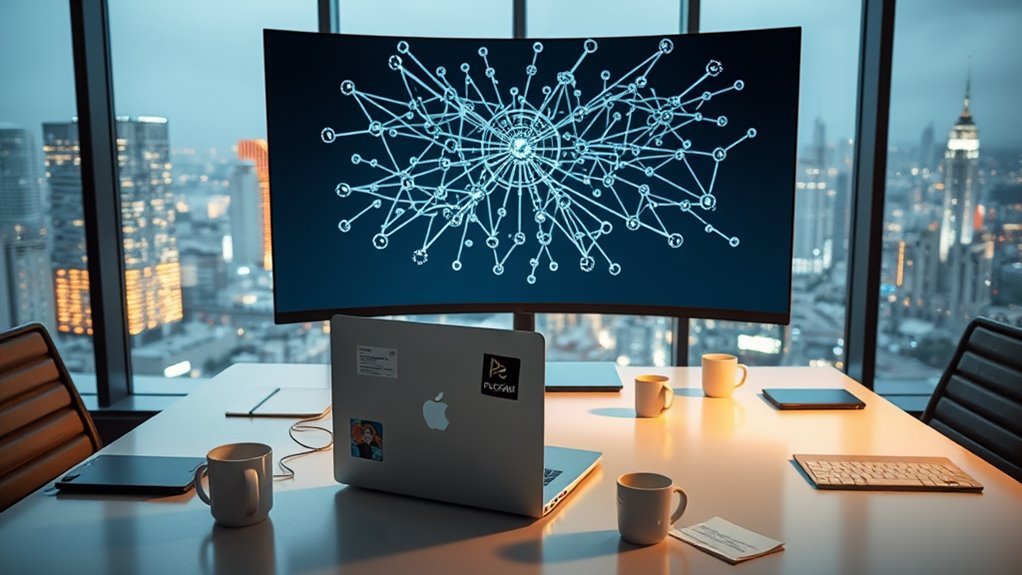
Utilize AI-driven task automation and orchestration to revolutionize your workflow by offloading repetitive processes to intelligent agents. This strategy allows you to tap into the immense potential of AI agents, which operate around the clock to manage tasks efficiently.
By adopting agentic workflows, you can achieve 24/7 operational capability, ensuring continuous productivity without downtime. Advanced AI agents utilize machine learning techniques, such as reinforcement learning, to adapt and optimize workflows dynamically based on real-time feedback and performance metrics. This leads to significant workflow optimization, resulting in improved operational efficiency.
Additionally, AI-driven task automation reduces human error by automating data-intensive tasks, ensuring higher accuracy and reliability in outcomes. The scalable nature of AI-driven task orchestration allows you to efficiently manage increased workloads and adapt to changing demands, thereby supporting growth and improving overall customer satisfaction.
Goal-Oriented Task Planning and Execution
By automating repetitive tasks, you've freed up resources to focus on more strategic initiatives. Now, it's time to optimize task workflow with goal-oriented task planning and execution. This strategy involves breaking down complex objectives into manageable subtasks, allowing AI agents to prioritize actions effectively and improve task execution efficiency.
Here are key aspects of goal-oriented task planning and execution:
- AI agents utilize decision-making algorithms to analyze data and generate potential actions, ensuring that selected tasks align with overall goals and maximize utility.
- Reinforcement learning techniques allow AI agents to adapt their strategies based on feedback from previous interactions, continuously refining their goal-oriented approaches for better outcomes.
- Integration of natural language processing (NLP) allows AI agents to understand user intents and contextual cues, enhancing their ability to plan and execute tasks that meet specific goals.
- Continuous monitoring and performance analysis support effective goal-oriented task execution, allowing AI agents to adjust their actions in real time based on evolving requirements and environmental changes.
- By leveraging these capabilities, AI agents provide actionable insights, helping you achieve complex objectives with precision and speed.
Adaptive Task Scheduling and Optimization
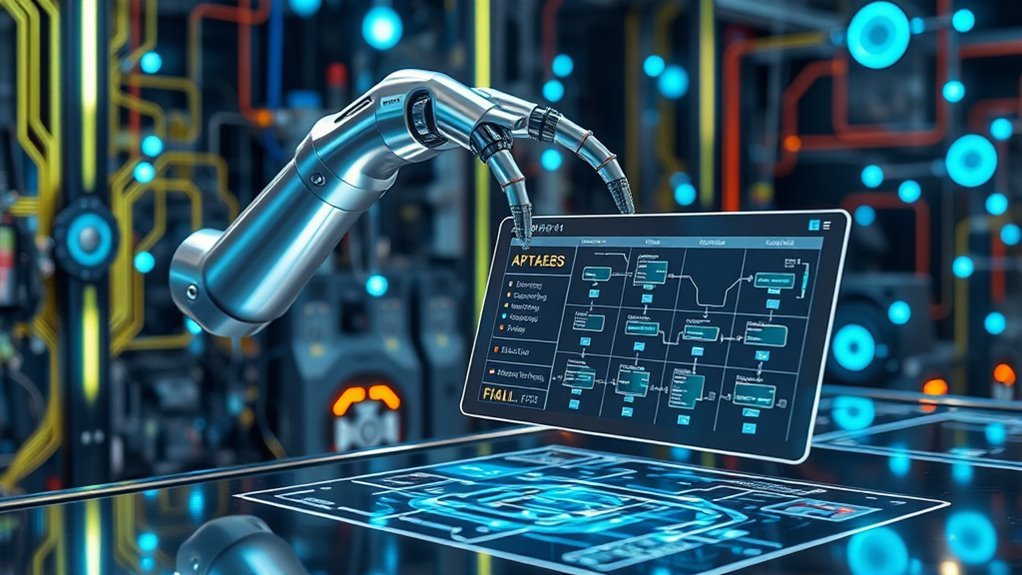
Effective task workflow management involves more than just automating repetitive tasks; it requires intelligent scheduling that adapts to changing circumstances.
With adaptive task scheduling, you can utilize AI algorithms to dynamically assign tasks based on real-time data, improving efficiency by up to 30% in workflow management. Machine learning models continuously analyze task completion patterns and resource availability, allowing for optimized scheduling that reduces idle time and boosts productivity.
AI agents can prioritize tasks by evaluating urgency, importance, and required skills, leading to a notable decrease in project delivery times by as much as 25%.
By integrating adaptive scheduling tools with existing project management software, you'll get a holistic view of task progress, facilitating better decision-making and resource allocation.
Furthermore, predictive analytics empower AI to anticipate potential bottlenecks in workflows, proactively adjusting schedules to maintain operational efficiency and meet deadlines.
Human-AI Collaboration for Task Completion
Cultivating a harmonious partnership between humans and AI agents revolutionizes task completion, as each entity focuses on its strengths to drive productivity. Through merging the strengths of both parties, you can create a workflow that's more efficient, effective, and adaptable.
Here are the benefits of human-AI collaboration for task completion:
- Automation and augmentation: AI agents automate repetitive tasks, freeing you to focus on strategic decision-making and creative problem-solving.
- Efficiency gains: Collaborative workflows can increase efficiency by up to 30%, as AI agents handle routine processes and you engage in higher-value tasks.
- Continuous improvement: Feedback loops between AI agents and human operators refine AI performance, ensuring that AI systems adapt and improve based on real-world interactions and outcomes.
- Seamless communication: AI agents with natural language processing capabilities facilitate communication with human team members, providing insights and recommendations that elevate collaborative task execution.
- Goal achievement: Organizations that employ AI-human collaboration frameworks are 40% more likely to achieve their project goals on time and within budget.
AI-Powered Task Analytics and Insights
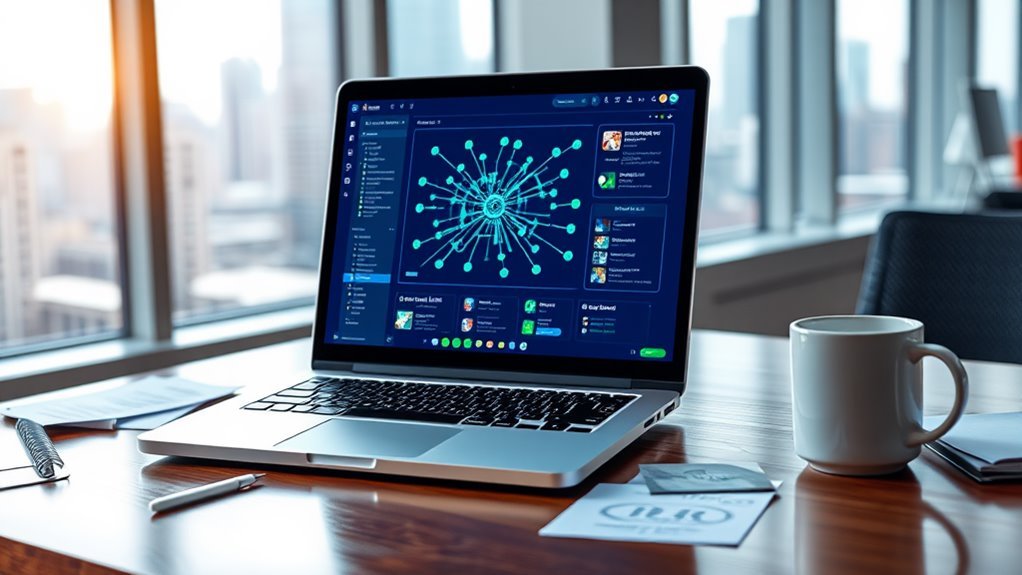
Within the domain of task workflow, AI-powered task analytics and insights emerge as a game-changer, empowering you to access the full potential of your data.
By leveraging advanced data processing techniques, you can derive actionable insights from large volumes of task-related data, ultimately improving decision-making and operational efficiency.
AI agents, equipped with machine learning algorithms, can identify patterns and trends in task performance, allowing you to optimize workflows and allocate resources more effectively.
Real-time analytics provided by AI systems can greatly reduce response times for task-related queries, enhancing overall productivity and customer satisfaction.
By incorporating AI-driven insights into task management, you can automate repetitive processes, receive data-driven recommendations for task prioritization, and increase efficiency by up to 30%.
With AI-powered task analytics, you'll gain a deeper understanding of your workflows, enabling you to make educated choices and drive continuous improvement.
Continuous Learning and Improvement Cycles
Your AI agent's performance improves dramatically when it's equipped with a continuous learning cycle. This iterative process refines decision-making by incorporating real-time feedback, making the agent more effective over time.
By leveraging reinforcement learning, your agent learns from its actions and outcomes, creating a feedback loop that drives adaptation and improvement.
You can implement a continuous learning cycle in the following ways:
- Utilize reinforcement learning to learn from actions and outcomes
- Establish a feedback mechanism from human users to refine AI agent behaviors and promote ethical decision-making
- Implement knowledge management systems to store and retrieve domain-specific information
- Regularly update models with fresh data to maintain relevance and accuracy
- Incorporate real-time feedback to augment decision-making and drive adaptation to changing environments
Most-Asked Questions FAQ
How Do I Ensure AI Agents Align With My Organization's Unique Needs and Goals?
You make certain AI agents align with your organization's unique needs by implementing customization strategies, engaging stakeholders, and setting clear goals; incorporate user feedback, iterative development, and performance metrics to secure goal alignment and adaptability.
What Are the Key Performance Indicators for Measuring AI Agent Success?
You'll want to track key performance indicators like task completion rates, response times, and user satisfaction to measure AI agent success, while likewise monitoring data accuracy, adaptability, and cost reduction to guarantee efficient and effective performance metrics.
Can AI Agents Handle Complex, Non-Routine Tasks and Decision-Making?
You're wondering if AI agents can handle complex, non-routine tasks and decision-making? While they struggle with adaptability challenges, human-AI collaboration and scenario-based training can help, but limitations in task automation and cognitive load management remain, requiring predictive analytics and real-world applications to overcome AI learning curves.
How Do I Address Potential Bias in AI Agent Decision-Making and Task Assignment?
You'll address potential bias in AI decision-making and task assignment by implementing bias mitigation strategies, such as ethical frameworks, diverse datasets, and transparency protocols, and continually plunging with feedback loops, algorithm auditing, and stakeholder engagement.
What Is the Ideal Human-To-Ai Agent Ratio for Optimal Task Workflow Efficiency?
You'll find the ideal human-to-AI agent ratio when you strike an automation balance, optimizing task allocation to maximize human productivity, while leveraging AI augmentation for efficient workload distribution, and monitoring efficiency metrics to guarantee seamless team dynamics and scalability potential.
Conclusion
You've now got the tools to revolutionize your task workflow with AI agents. By implementing these 10 strategies, you'll be able to prioritize tasks more efficiently, allocate resources dynamically, and automate mundane tasks. With real-time monitoring and human-AI collaboration, you'll be able to optimize task completion and gain valuable insights. Continuous learning and improvement cycles will guarantee your workflow stays ahead of the curve. It's time to unleash the full potential of your task workflow with AI agents.